solution
Increased nitrogen loading to rivers due to human activities was blamed for the rise in abundance of algae in coastal waters. For example, the Neuse River Estuary fishkills in the 1990s were attributed to the increased algae due to increased nitrogen loading from the Neuse River basin, especially from concentrated animal farming in Eastern North Carolina. Conclusions like this are often made from analyzing crosssectional or metadata. Cole et al. [1993] assembled a data set including many large river systems in the world to study the effect of human activities on nitrogen loadings to rivers (data file nitrogen.csv). They included nine variables in the data set: (1) discharge (DISCHARGE), the estimated annual average discharge of the river into ocean (in m3/sec); (2) runoff (RUNOFF), the estimated annual average runoff from watershed (in liters/(sec×m2 )); (3) precipitation (in cm/yr) (PREC); (4) area of watershed (in km2 ) (AREA); (5) population density (in people/km2 ) (DENSITY); (6) nitrate concentration (in µ mol/L) (NO3); (7) nitrate export (EXPORT), the product of runoff and nitrate concentration; (8) deposition (DEP), nitrate loading from precipitation – product of precipitation and precipitation nitrate concentration; and (9) nitrate precipitation (NPREC), the concentration of nitrate in wet precipitation at sites located near the watersheds (in µ mol/(sec×km2 )). In the paper, the authors used nitrate concentration (NO3) and nitrate export (EXPORT) as measures of human impact on rivers. The authors looked at these two response variables separately to determine whether the impact of human activities in river nitrogen level is a result of direct pollutant discharge input to the river or discharged indirectly through atmospheric pollution. They suggested that population density in the watershed can be used as a measure of direct discharge and nitrate precipitation can be served as a measure of indirect discharge.
Fit a regression model for each of the two response variables and discuss whether anthropogenic impact of river nitrogen is more through direct discharge or through indirect atmospheric deposition. Note that the two factors are correlated and their effects are unlikely to be additive.
"Looking for a Similar Assignment? Get Expert Help at an Amazing Discount!"
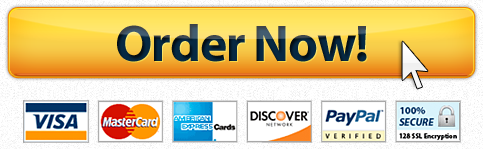